Neural Network World is a bimonthly journal providing the latest developments in the field of informatics.
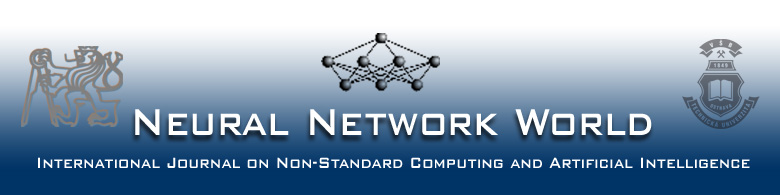
The journal devotes its attention dominantly to the problems of:
- brain science,
- theory and applications of neural networks (both artificial and natural),
- fuzzy-neural systems,
- methods and applications of evolutionary algorithms,
- methods of parallel and mass-parallel computing,
- problems of soft-computing,
- methods of artificial intelligence
Announcements
Editorial: Editors' message to authors. |
|
Dear authors, a queue of hundreds of papers is awaiting processing (mainly for review). The procedure is longer and more complicated if the papers are not in the expected format. Please, respect the required paper format and formal requirements, otherwise, your manuscripts will not be accepted or their processing will take a prolonged time. | |
Posted: 2025-02-26 | |
Editorial: Announcement of charge imposition |
|
For manuscripts submitted after 2016/01/01, a new rule is coming into effect, namely an imposition of a charge on all published manuscripts. More information can be found below. | |
Posted: 2015-12-16 | More... |
More Announcements... |