Neural Network World is a bimonthly journal providing the latest developments in the field of informatics.
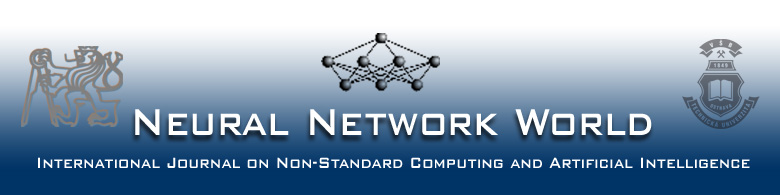
The journal devotes its attention dominantly to the problems of:
- brain science,
- theory and applications of neural networks (both artificial and natural),
- fuzzy-neural systems,
- methods and applications of evolutionary algorithms,
- methods of parallel and mass-parallel computing,
- problems of soft-computing,
- methods of artificial intelligence
Announcements
Editorial: Announcement of charge imposition |
|
For manuscripts submitted after 2016/01/01, a new rule is coming into effect, namely an imposition of a charge on all published manuscripts. More information can be found below. | |
Posted: 2015-12-16 | More... |
More Announcements... |